It starts with a cautionary tale.
Back in 2012, JP Morgan Chase’s report on their $6 billion trading loss revealed issues with the Value at Risk (VaR) model, indicating that volatility was lower than it should have been, in part, due to VaR having been calculated with Excel spreadsheets.
Ten years on and similar mistakes are still being made. An incorrect model at Silicon Valley Bank led to the second largest financial crisis since 2008, with root causes including lack of integrated modeling and limited risk management due to siloed spreadsheets on desktops. The bank collapsed on March 10, 2023.
Spreadsheets are so widely used, they’re a hard habit to break. But factors such as continued market volatility are raising the stakes to change. The hospitals experiencing massive labor shortages, hotels industry put on hold by the COVID-19 pandemic, carmakers hamstrung by global microchip shortages have keenly felt the effects of volatility through widely fluctuating business factors.
Scenario modeling shows how a business will respond to different sensitivities, enables teams to test hypotheses before budgeting and planning and better assess risk, but altering multiple sensitivities and tracking the corresponding outcomes is time-consuming, prone to errors, and almost impossible to audit.
For this reason, many finance leaders are turning to low-code/no-code data science platforms, which are designed to handle complexity and reduce error-prone manual work.
Equipped with advanced analytics and machine learning algorithms, these platforms are built to perform complex, compute-intensive analytics, handle huge datasets, and automate repetitive tasks. The intuitive interface lowers the entry barrier to advanced analytics capabilities for all, enabling users to build and deploy financial models quickly by drag and drop.
With the analytic breadth and depth of a no-code/low-code data science platform, finance teams can connect to all the relevant data, test out multiple what-if scenarios, and ensure their forecasts are as well-informed as possible.
Well-informed financial models built with advanced analytics
The table below shows a checklist of basic through to the advanced analytic capabilities for well-developed financial models.
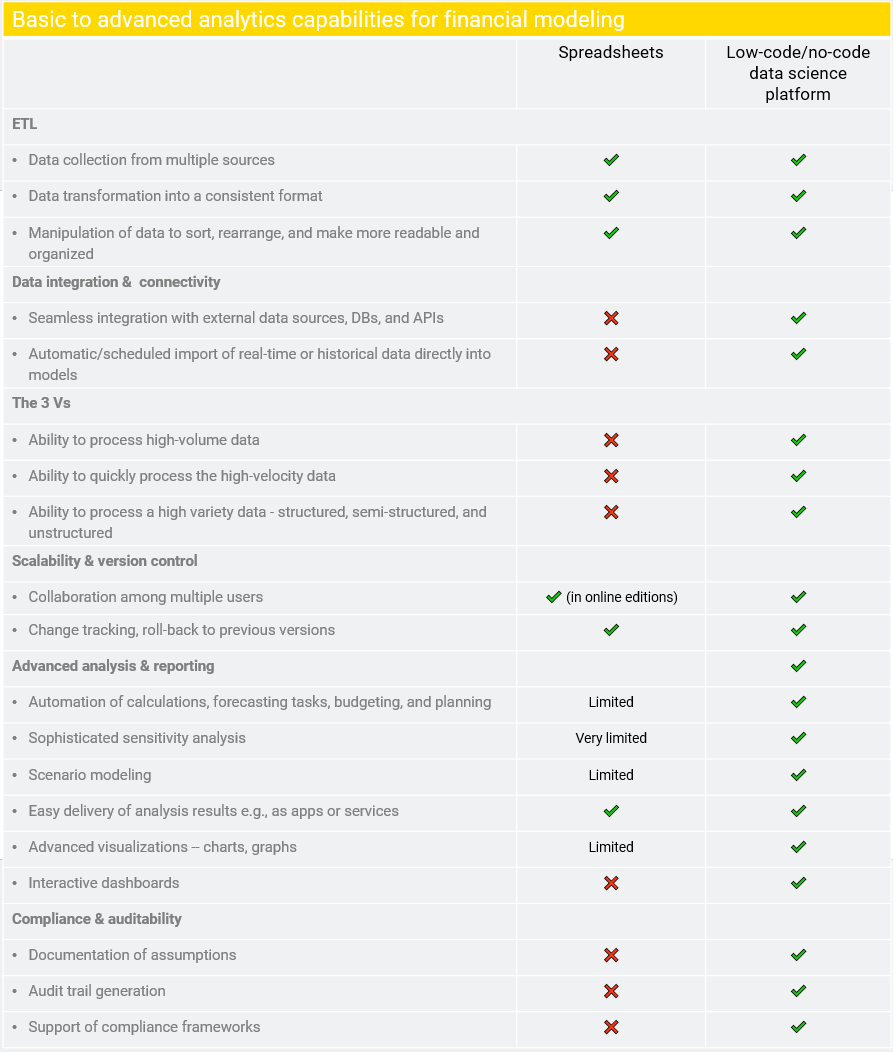
Let’s look now at five examples of how data science techniques enable more efficient, reliable, and auditable modeling.
1. Evaluate multiple hypotheses and gauge impact within seconds
Sensitivity analysis in spreadsheets becomes error-prone when the model is complex and requires entry of multiple formulas and variables.
Using a low-code/no-code data science tool, teams can run 10 different scenarios, set parameters in 10 different ways, assess the impact of changes in sensitivities, and get an evaluation and a visualization within seconds. For example, with topline revenue and bottomline profit assessed quickly for each scenario, finance teams can provide meaningful insight into gauging the effect of each “what-if” and prepare better for potentially difficult future scenarios.
2. Automate calculation and testing of scenario modeling and reduce human error
Keying in the wrong formula or copying over the incorrect amount are inevitable when data entry is manual. Automation reduces human error and gives teams time back to use their expertise where it’s most valuable, namely evaluating “what-if” scenarios, rather than checking spreadsheets.
Scenario modeling itself can also be automated to run e.g. once a quarter, with the results incorporated into the organization’s rolling forecast. This ensures the forecast takes all business changes into account.
3. Develop explainable models and gain full auditability
Financial loss, regulatory issues, and damaged reputations are some of the repercussions from inaccurate credit scoring models. Understanding the logic and flow of calculations can be difficult, especially for complex models created by others. This lack of transparency makes it challenging to verify and review the model's accuracy and reliability.
Self-documenting solutions provide a full capture of a solution’s metadata. Regulatory agencies can track and verify each data point back to its source. Incorrect data or calculations, unauthorized access or changes to critical data are captured and flagged. In addition, explainable AI methods can be used to understand how a machine learning model is computing outcomes.
4. Deal elegantly with data volume & variety for comprehensive risk assessments
As a finance leader ten years ago, you only worried about financial data. New legislation, for example, in the EU requires financial market participants to show how ESG risks are integrated into decision-making. But ESG data is not standardized or as readily available as financial data. It’s unstructured data, tricky to collect and tricky to process.
Low-code, no-code data science tools deal elegantly with both structured and unstructured data, with efficient data transformation techniques to bring it into a consistent format for analysis. Models for ESG risk can then be trained on the company’s financial and non-financial data. Running scenario simulations is performed quickly enabling efficient analysis of extensive financial data and large-scale scenarios.
5. Analyze real-time data and remain nimble to change
By importing real-time data directly into financial models, teams remain nimble to changes in the data. As market volatility increases, so can fraud, reported FINRA. Criminals are persistently finding new ways to circumvent measures put in place to prevent fraud, making their activities difficult to detect.
Utilizing real-time data and automating the frequency of building forecasting models based on this data enables teams to test out unlimited scenarios to make their budgets and forecasts as well-informed as possible. Data mining techniques reveal patterns in financial data not possible with spreadsheets, enabling finance teams to “better detect suspicious transactions and customer behaviors”.
Equip your team with data science skills for reliable financial modeling
Your finance team is involved in every department, business unit, and subsidiary, gathering and analyzing data to get the insight you need for the recommendations you, as a finance leader, bring to your CEO’s inner circle. It’s time to level up your team’s data science capabilities. With these skills they’ll be able to develop up-to-date models, and be ready to point to exactly the line in the very complex report that justifies a decision.
It’s time to break the spreadsheet habit.