A fire is never a welcome occurrence, but having a responsive fire department can make all the difference. In the Netherlands, the standard dictates that the fire department should arrive within 8 minutes of receiving a call, a crucial factor in minimizing damage and ensuring safety. However, what happens when this response time is jeopardized by the closure of a fire station?
Geospatial analysis is invaluable at uncovering the real-world implications of operational decisions. However, accessing and analyzing geospatial data has traditionally required specialized expertise, which has blocked geospatial data’s full utilization.
This article (a revised version of the original post published in Low Code for Data Science) explores the implications of such an event and how you can use geospatial analysis to uncover the impact on community safety. I’ll show how I analyzed geospatial data using the low-code tool KNIME Analytics Platform. KNIME’s low-code interface allows users to easily load and manipulate geospatial data; it makes geospatial analysis available to all.
Task: Assess the impact of fire station relocation using geospatial analysis
On January 1, 2023, the closure of a fire station in my hometown marked a significant change in the emergency response landscape. To understand the full extent of this shift, I set up an analysis focused on one central question: What is the travel time from the fire station to every street in the affected area? Armed with data and KNIME, I set out to reveal the implications of this operational change.
Analyze geospatial data using KNIME in 4 steps
As is often the case, I conduct my analyses in KNIME. The Geospatial Analytics extension enables you to not only create beautiful visualizations (which speak louder than 1000 words), but also perform all sorts of geospatial calculations and visualizations.
The geospatial nodes work very well with the OpenStreetMap integration. Let’s look at the four steps it took to reveal the shift in travel time from the fire station to each street in the affected area, and how I built this workflow.
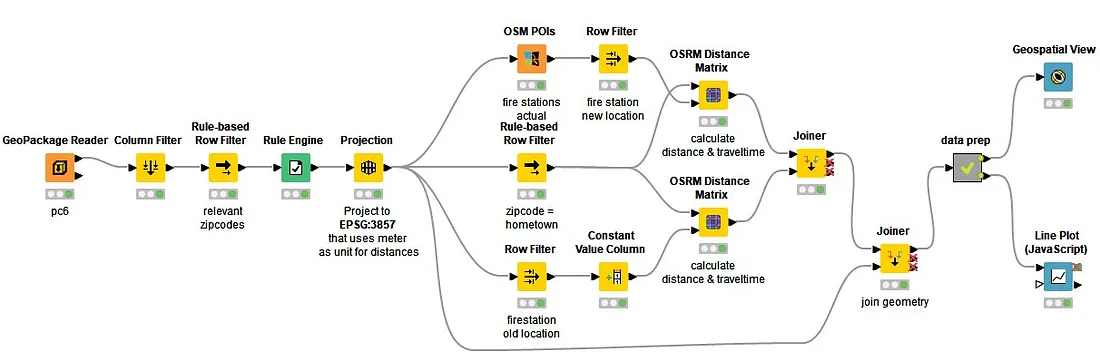
Step 1. Build the foundation for the map
To build the foundation for a map, I needed a file containing the geographical areas of all zip codes in my hometown. Each zip code covers a part of a street with an average of 25 addresses (residential and/or commercial properties). A complete file of the Netherlands containing this information (and many more features) could be downloaded from the CBS website.
The first step is to read in this downloaded file. This is easily done with the KNIME GeoPackage Reader. All you need to configure is a link to the file on your computer. It is quite a large database, so I used a Row Filter node to select only the necessary zip codes, and with the Column Filter node, I ensured that I retained three columns (geometry, postcode, number of inhabitants).
To accurately calculate the distance and travel time between the fire station and alle the streets later on, a projection from the current Coordinate Reference System (CRS) to the new CRS (epsg: 3857) was necessary. This new projection is a Web Mercator projection used by many web-based mapping tools, including Google Maps and OpenStreetMap.
Step 2. Find geolocations with Open Street Map POIs in KNIME
In this step, I searched for the address of the old fire station and the location of the new fire station. You can do this by googling. But I went for the OSM POIs node. I find this KNIME node super handy.
Objects within OpenStreetMap such as shops, bus stops, and statues are assigned one or more keys with a value.
So, for all objects you encounter on OpenStreetMap, you can retrieve their geolocation and other useful meta information.
You can find for example all fire brigade stations in your dataset (with a geometry identifier) by searching for key = ”amenity” and value = ”fire_station”.
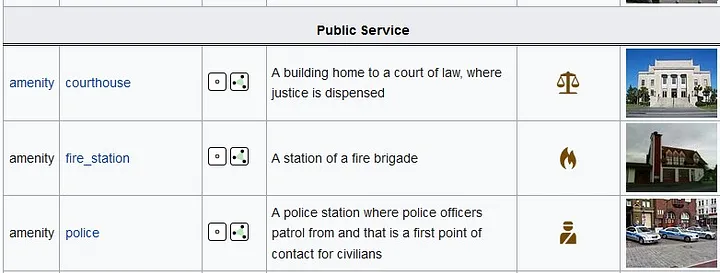
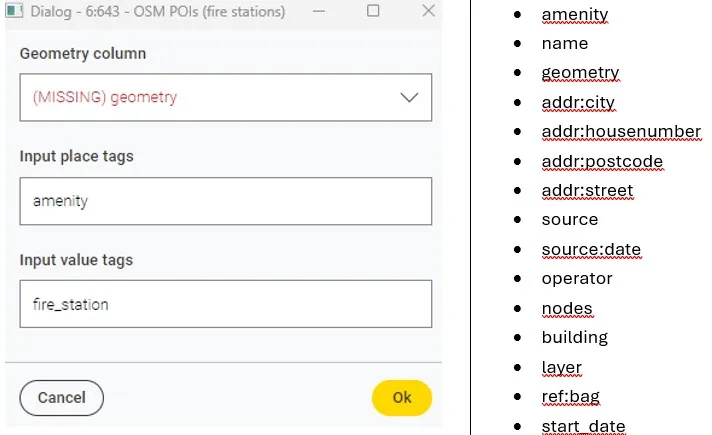
Step 3. Calculate distances and/or travel time
Now I have the geolocations of the fire stations and all the streets available. I used the OSRM Distance Matrix node to calculate the distance (in meters) and/or travel time (in minutes) and/or route.
This node uses the Open Source Routing Machine (OSRM) to create a distance matrix for the provided origins and destinations.
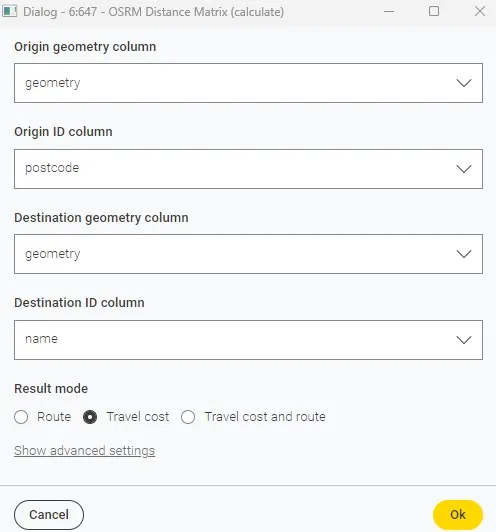
Step 4. Create a map to show travel time from new fire station to streets
With all this information available, I’m able to create a map, showing the duration to drive from the new fire station to every street.
The configuration of the Geospatial View is straightforward, the result impressive (check out the Base Map Setting in the configuration tab).
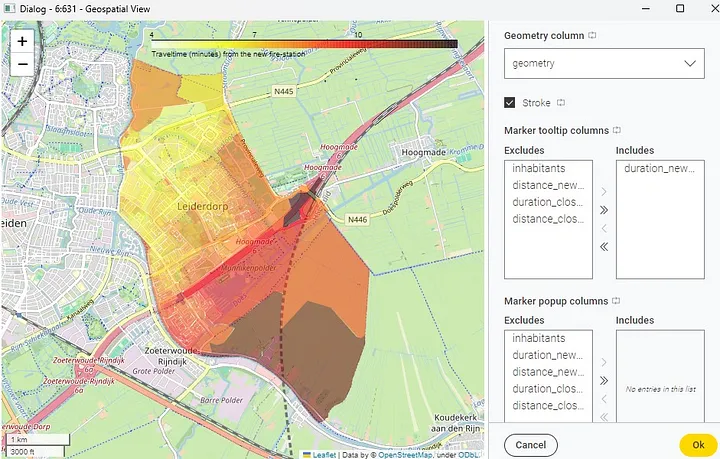
Results: 22% of residents now over 8 minutes from nearest fire station
The findings were revealing. It became apparent that approximately 22% of my hometown residents now reside more than 8 minutes away from the nearest fire station, a stark contrast to the previous scenario where everyone was within the prescribed response time.
This shift, particularly affecting those in the southern part of the town, underscores the tangible impact of operational changes on community safety.
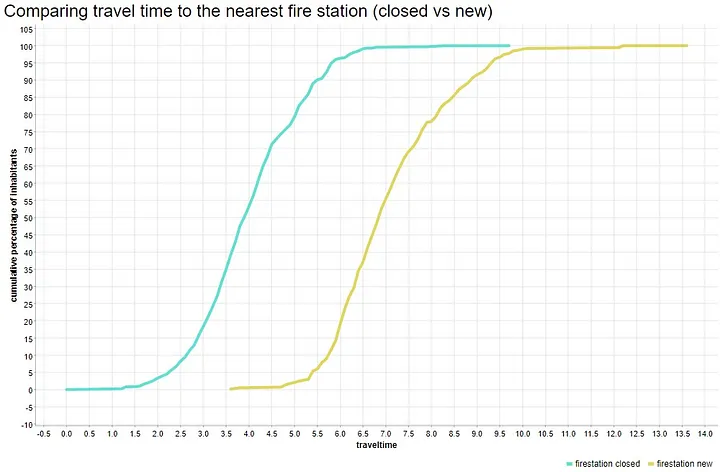
Try geospatial analysis yourself
Geospatial analysis highlights the critical role insight can play in informing policy, planning, and, as I’ve shown here, ensuring the safety and well-being of communities.
The insights of the impact of relocating the fire station is just the tip of the iceberg of what you can achieve with the Geospatial nodes in KNIME. There is so much more to discover and create with the 96 nodes of this Geospatial Analytics extension.
I would say, don’t wait for my next blog post, explore the practitioner’s guide to gespatial analysis, and get started yourself!