Generative AI has upped the stakes for anyone who cares about data privacy and model governance. It’s hard enough to govern data and analytical models within a closed environment. But the breakneck emergence and adoption of Generative AI tools has made it tough for anyone to keep up and effectively govern its usage. To be fair, data privacy and model governance are not new topics. But GenAI has opened up a new dimension of complexity to these issues.
According to Cisco, 27% of companies have chosen to manage the risk by banning GenAI tools outright. Early and highly public blunders, like Samsung staff leaking proprietary code to ChatGPT, don’t help either. This is just one example of many issues that have raised concerns in the data science community about how to create and uphold a watertight data and model governance framework while still reaping the benefits of GenAI.
Even before GenAI, we needed to govern which models we provide access to (and for what), who gets to work with these models, and which data is safe to share. That’s all before even thinking about adding GenAI to the mix. Now we have to manage risks to ensure none of our data gets sent where it shouldn’t and that our models don’t produce unethical and incorrect results.
In this article we’ll look into how you can balance the benefits and risks of GenAI in the context of data science without resorting to blanket bans.
Risks and opportunities of GenAI for data scientists
The emergence of GenAI thrust many chief data officers into the spotlight, as the rest of the C-suite asked “how do we manage all the risks from GenAI”, and looked to data scientists and data stewards for answers.
Before we can manage risks, we need to know what those risks are. The first step to creating a strong data science governance approach for GenAI is appraising the benefits and risks and making sure your actions and policies reflect your own risk tolerance. So let’s take a look briefly at the benefits and in more detail at the risks.
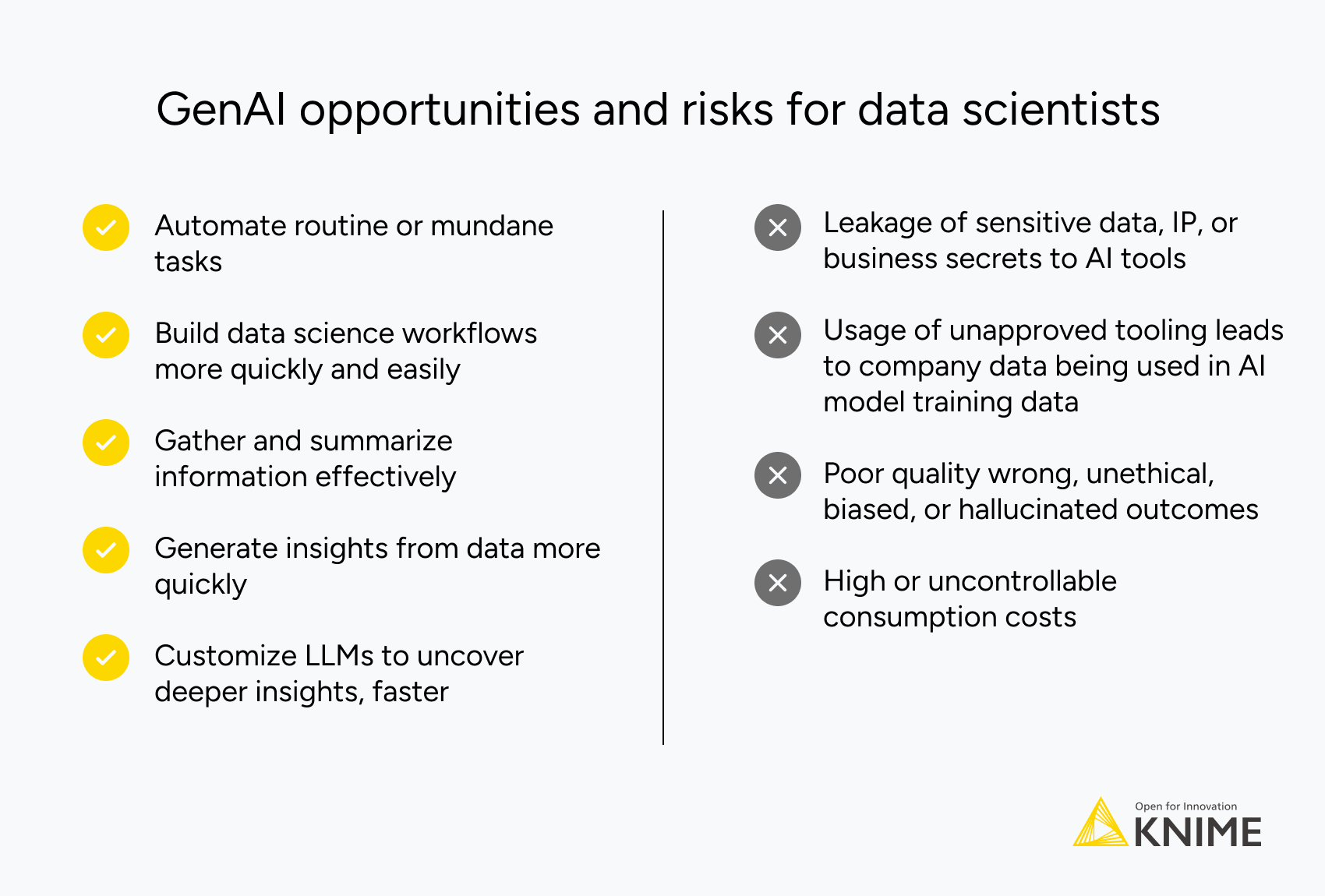
How data scientists benefit from GenAI
Generative AI has not and will not replace data workers, even if that claim makes for a good piece of clickbait. It has already proved helpful to automate a lot of the mundane tasks data workers need to take care of, and we will likely see even more automation benefits as the technology develops.
Today, preparing data, cleaning data, and even building basic workflows can be taken care of by GenAI. And beyond just efficiency gains, it gives time back to our data experts to undertake more complex and creative work and spend less time on the boring but necessary things. Even beyond the mundane “helper work”, we can now incorporate large scale GenAI models into our analytical work easily to expand the scope of what we’re able to achieve.
And this is only the beginning.
We can expect the capabilities of GenAI to improve even further over the coming years in ways we are not even able to foresee. Can our approaches to GenAI governance keep up?
How data scientists face risks from GenAI
Data governance risk
Data governance and data security are two of the biggest concerns about rolling out GenAI usage across enterprises. There are very real risks that proprietary or personal data is accidentally leaked to AI tools and then used to train models or exposing companies to the risk that a third-party can read and analyze data sent to these tools.
Model routing and consumption risk
GenAI brings with it risks around cost control, reliability of models, and the internal risk of people using unapproved tools. When organizations have no policy around ruling in or out specific GenAI providers, they can end up in a situation where people across the company use countless different GenAI tools. This can lead to data privacy risks as data gets sent to multiple tools, but also consumption risk, as you may find yourselves racking up big bills with various providers.
Model quality risk
With GenAI tooling, we need to ensure output quality. That means building in checks and balances to identify hallucinations, inaccuracies, and bias in outputs. There have already been high profile cases and studies in which these risks have been identified:
- In 2023, two lawyers were fined $5,000 for using ChatGPT to draft a court filing that they failed to independently assess the accuracy of. The legal precedents presented by ChatGPT in the court filing turned out to be wholly inaccurate.
- According to an analysis of over 5,000 Stable Diffusion images, researchers showed that “The world according to Stable Diffusion is run by White male CEOs. Women are rarely doctors, lawyers or judges. Men with dark skin commit crimes, while women with dark skin flip burgers.”
GenAI can cause not only reputational damage but also costly legal liabilities if the output is not thoroughly checked either by humans or by systems that will flag problems.
How to start managing the risk of your GenAI usage
Effective governance does not involve only setting prohibitions and locking functionality away. It’s about finding balance and making smart choices. It’s about asking how we can make the most of the powerful generative AI technologies entering the market while keeping our data secure and safeguarding against risk.
As a low-code tool for data scientists, data wranglers, and data analysts, KNIME users can process a lot of potentially sensitive data to run complex analyses. KNIME users can build GenAI capabilities into their visual workflows to augment their analytics. This means users can use GenAI for content summarization, domain knowledge extraction, or any other LLM capability. Mixing LLM capabilities together with KNIME’s other analytics and visualization techniques enables users to further customize and expand the boundaries of their data work. What’s more, KNIME’s GenAI chatbot K-AI can upskill your teams quickly and help you build basic workflows that give you a head start.
These benefits come with all the risks we’ve discussed around hallucination, model governance, and bias – but can be governed with the enterprise capabilities available in KNIME Business Hub. Let’s take a deeper look at how you can govern your GenAI usage with these features.
How to manage model routing risk for GenAI
Beyond domain restriction on your company network or machines, it’s not always easy to lock-in a “preferred” or “trusted” AI provider for your company. In an organization of hundreds or thousands of people, how can you be sure your data scientists aren’t using unapproved GenAI tooling?
With KNIME, administrators can set one or more proxy GenAI provider for data scientists. This way, you can be sure analytical workflows are only interfacing with trusted and vetted GenAI tools. This puts the power in your hands and gives you some peace of mind that your GenAI governance framework is adhered to.
You can also set permissions for different AI processors for different teams. And you can even point your proxy to an in-house model as well if, like Samsung in our example above, you want to build or use an internal AI tool instead.
How to manage data governance risk for GenAI
Limiting this risk of data leakage begins with having a solid internal data governance and access hierarchy. Only the relevant people should get access to the relevant data they need to be effective, and at the appropriate level of abstraction. This step alone can go a long way to minimizing risk of data leakage to GenAI tools.
Even if we think we’ve removed all personally identifiable information from datasets, we can never entirely remove human error. There’s always a risk that someone in your organization could run an analysis with sensitive data that is sent to your approved GenAI provider’s servers. But we can set checks and balances to detect that human error before it’s too late.
With KNIME, you can set guardrailing workflows that will take care of anonymizing data or block any workflows that include, for example, PII. This adds an additional layer of protection that stops data being read by an AI and protects you from embarrassing mistakes.
How to manage model quality risk
Artificial intelligence is incredibly helpful, but it does not replace natural intelligence. When it comes to evaluating the accuracy of GenAI output, the best way to do this is to manually review output and always have a human in the loop reviewing them.
With KNIME, administrators are able to look back at a workflow that produced an inaccurate result and access logs that show which validation workflows were triggered (e.g. the PII anonymization workflow, or specific workflows that block access to GenAI tools). Data scientists and stewards can then use these insights to amend or improve their governance approach.
KNIME’s visual workflows also allow you to easily see all the steps in a data science workflow. In the case that something does go wrong with your GenAI tooling, you are able to visually inspect your KNIME workflow and in particular review the prompts used so you can get a complete picture of what happened.
The path forward for data science and GenAI
Those of us working in data compliance feel the risks of GenAI technologies all too keenly. The difficult thing is finding the right balance of checks and controls to make it feel “safe enough”. Recent data shows that 91% of organizations feel they need to do more to reassure customers about the use of their data with AI tools and 92% believe that GenAI requires a fundamentally different approach to governance.
There will never be a 100% security guarantee with GenAI tools unless you host them locally. But with the right safeguards we can protect ourselves and better balance the risks and benefits of this new technology.
At KNIME, we’re creating an environment where you have the flexibility to make those choices for yourself – no matter your risk tolerance. And as GenAI technologies evolve, so will we.