Disclaimer: While KNIME Analytics Platform is free and open source, executing the below-mentioned workflows, available via a third-party extension, require a commercial cloud service.
A significant 77% of marketing ROI comes from segmented and targeted campaigns. Customer segmentation is an essential business strategy used to better understand the customers, their demographics, behavior, and needs. But traditional customer segmentation techniques lack the sophistication to provide customers with the personalized experience they demand today.
Hyper-segmentation, on the other hand, takes customer segmentation to the next level by creating very specific and narrow segments based on detailed customer characteristics. It enables businesses to develop highly targeted and personalized marketing campaigns, leading to increased customer engagement and loyalty.
Hyper-segmentation has some challenges. It requires analyzing large volumes of customer data, which can be costly and time-consuming and it involves identifying very specific and nuanced customer characteristics, which may require domain-specific knowledge and expertise. AI applications can help overcome these challenges by providing data scientists with advanced tools and techniques to automate the process of data analysis and identify complex patterns in customer behavior more efficiently, accurately, and within minutes, saving costs and time.
Integrate know-how from subject-matter experts for greater business insight
Even greater insight can be revealed by AI applications when subject-matter experts are enabled to inject their knowledge into the application. Here we show how, with the Symanto Brain extension for KNIME.
Symanto Brain enables subject-matter experts to easily and efficiently build their own text analytics models within KNIME in only a couple of minutes. It includes advanced natural language processing techniques and machine learning algorithms that allow you to extract insights from your text data and make data-driven decisions. Together with KNIME Business Hub, companies can leverage applications of these models across the business.
Hypersegmentation with the help of AI
In order to have a successful hyper-segmentation, we will follow 5 pre-defined steps which cover the whole process of data collection to the visualization of the end results.
To help you perform the segmentation, you can download this example workflow that covers steps 2 to 5 from below.
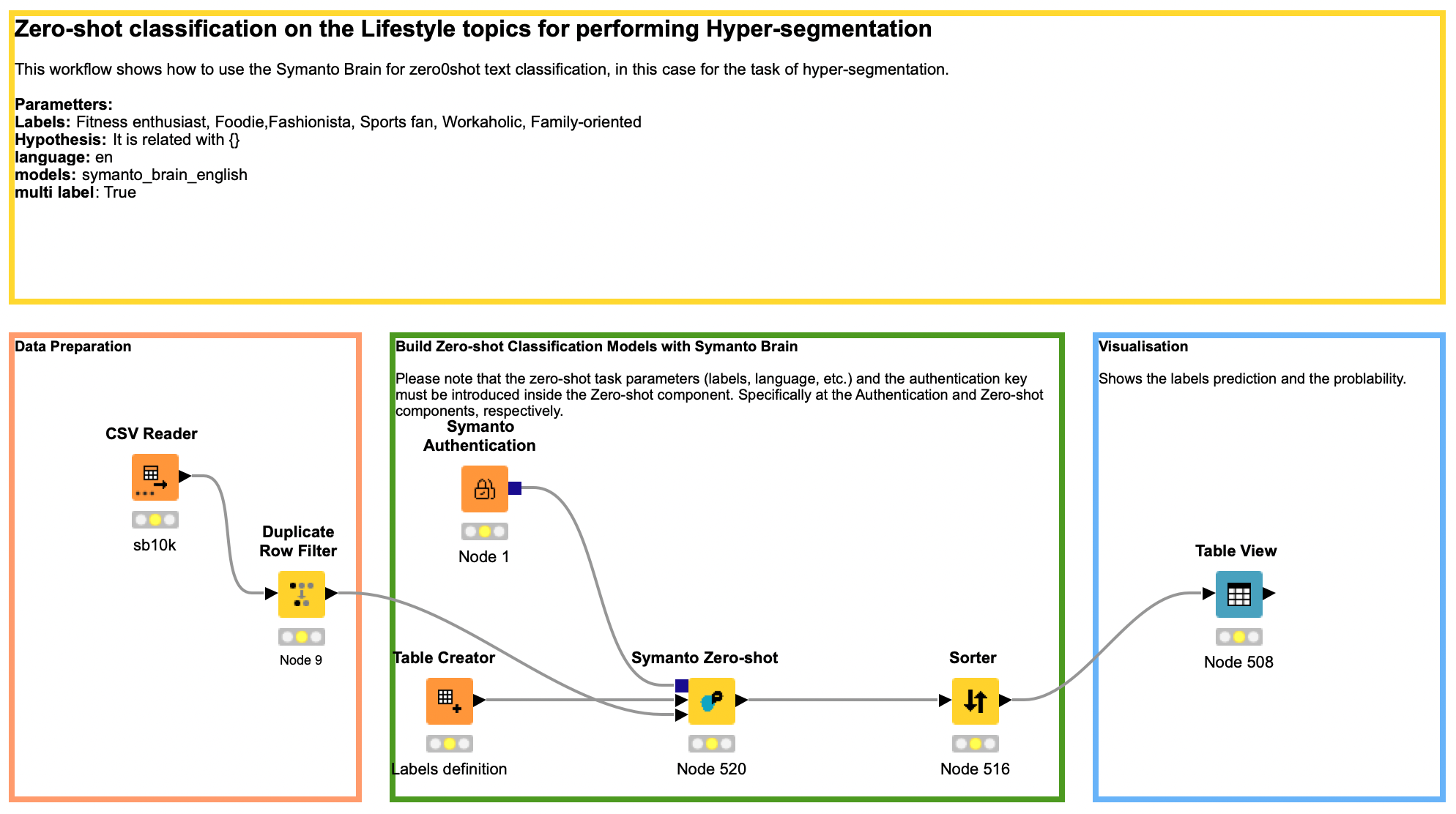
Step 1: Collect data
The first step is to collect customer data. This can include demographic data, purchase history, social media activity, and more, and can be collected through various methods, including surveys, customer feedback, and social media monitoring.
The more data is collected, the more specific the hypersegmentation will be.
Step 2: Prepare the data
The next step is to prepare the data for analysis. Clean the data, remove any duplicates, and standardize it to ensure that it is consistent and accurate. This step is critical to ensure that the text analytics models are effective in identifying patterns and trends in the data.
Step 3: Choose subsegments for your models
With the data prepared, you might already have an idea of what types of segments you would like to discover and learn more about. Each segment will then require its own unique text analytics model. In order to create one, it is necessary to define the classes or labels that the model will use to classify the text accordingly. In this case, these labels will be the sub-segments.
Here are some examples of specific and narrow segments for which you can build models to segment the customer data and example labels that you might use for them:
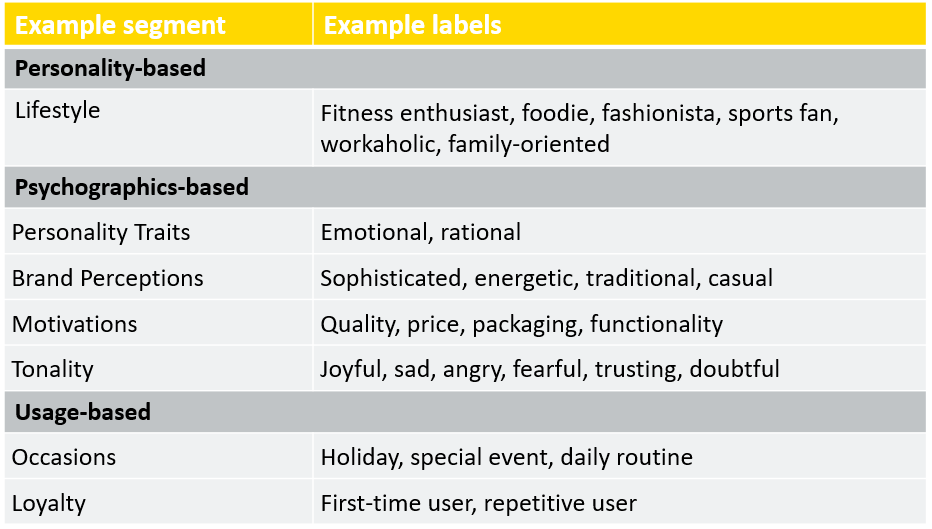
Step 4: Create the text analytics models
Once your data has been prepared, you can proceed to create your models by installing the Symanto Brain extension.
This extension is equipped with a comprehensive, in-house pre-trained Transformer model, advanced NLP techniques, and machine learning algorithms that enable you to create your models quickly and easily in just a matter of minutes.
To use the Symanto Brain extension, you need an API key:
-
Drag and drop the Authentication node.
-
Right-click and select configure.
-
Add your API key. If you don’t have one, you can navigate to the Symanto Developer portal by clicking the Get API key button, sign up and then get the free API key from Subscriptions in your Profile section.
-
Click the Apply button. If all settings are valid, the node status will change to configured.
-
Right-click and execute the node.
-
Once you are authenticated, add a Table Creator to define your labels:
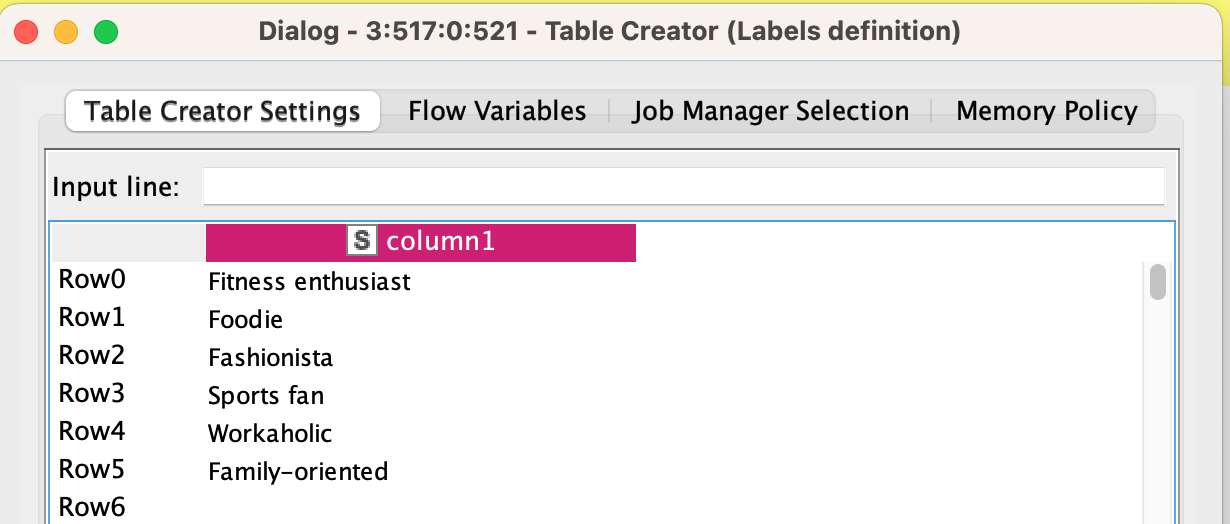
Once you have the labels and the input data, you can start creating your model:
-
Drag-and-drop the Zero-shot node.
-
Right-click and select Configure. A dialog window opens to select the key elements to configure your model:
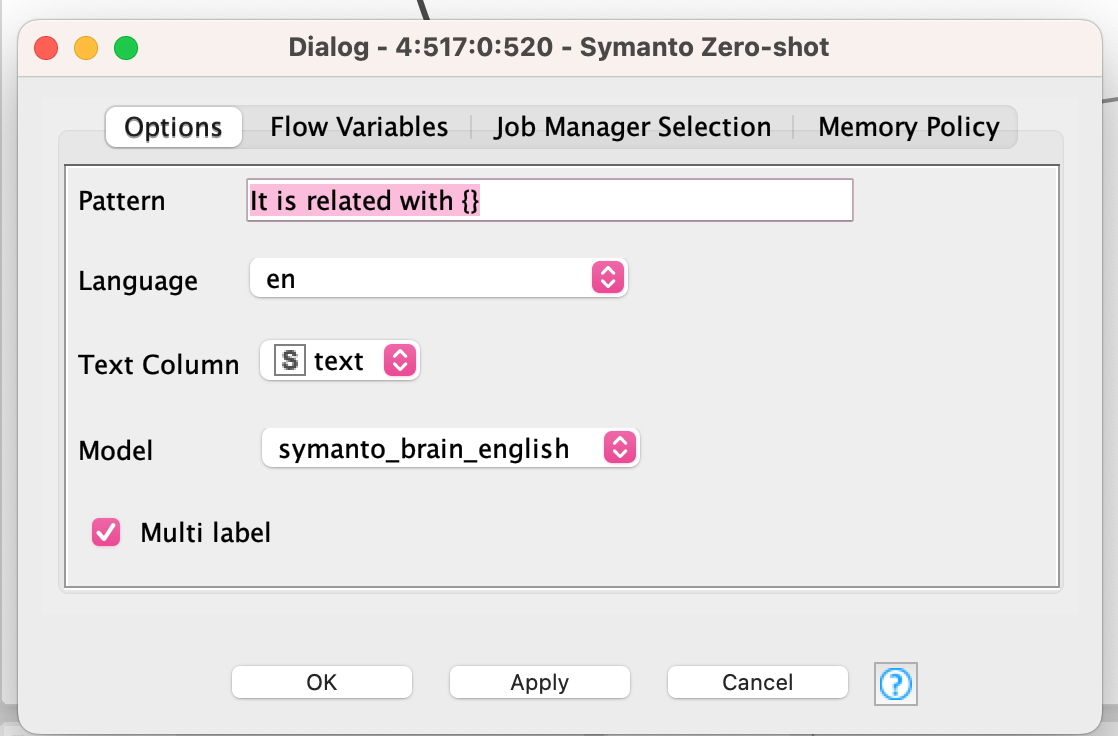
-
Select the five key elements:
-
Patterns, known as label descriptors, provide context to the model and allow the semantic matching between the analyzed text and the
-
Your labels, e.g. This text is {} or This person talks about {}
-
Language, i.e. the language your text is written in. You can select it from the drop-down menu.
-
Text column, i.e. the field which will be considered as text in your data file.
-
Model, i.e. the embedding model that will be used.
-

-
Check "Multi label" if you want your texts to be classified with multiple labels (sub-segments)
-
Click the Apply button. If all settings are valid, the node status will change to configured.
-
Right-click and execute the node.
-
When the status is changed to Executed , the Zero-shot node will calculate and produce a table.
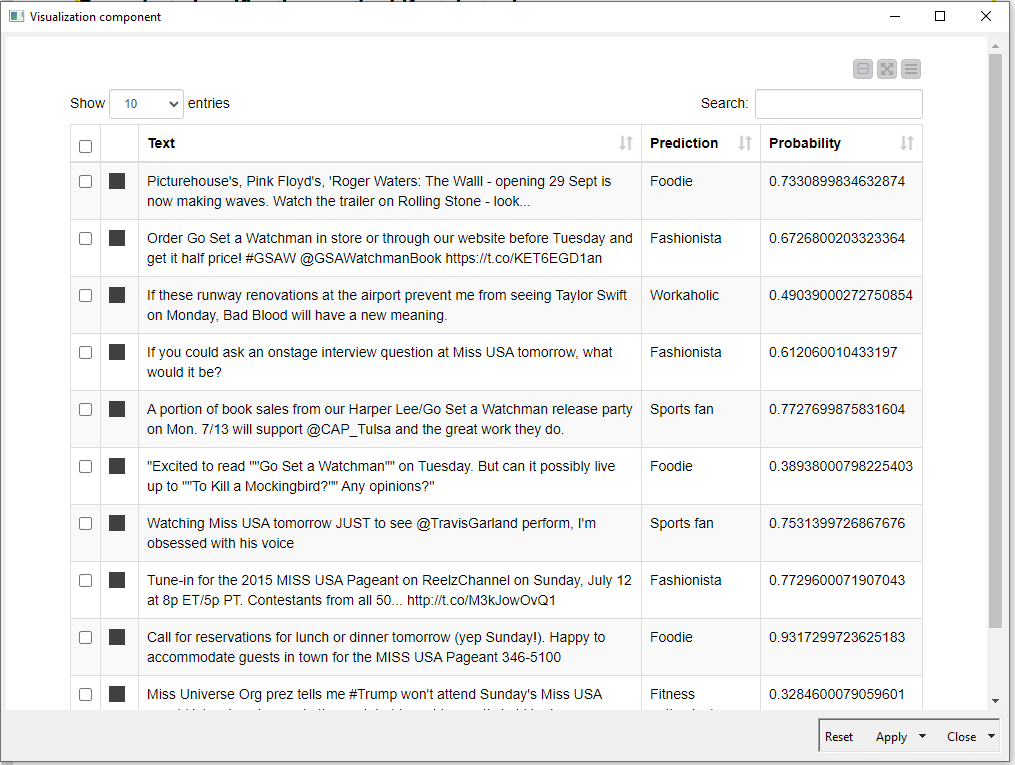
Step 5: Results and visualization
After applying the text analytics model to your data, you can see how it is segmented based on the specific task you wanted to accomplish using KNIME's built-in visualization tools. By visualizing the results you can gain a deeper understanding of the patterns and trends in your data, and communicate these insights to others in your organization. This can help you to create more targeted and personalized marketing campaigns since the high level of personalization can significantly improve customer engagement and loyalty and increase business performance overall.
Deploy analysis across the enterprise with data apps and services:
Hypersegmentation and AI have the potential to provide businesses with valuable insight for more informed, personalized, and targeted marketing campaigns to improve customer engagement and loyalty and efficiently drive sales and revenue growth.
Organizations can scale the impact of this insight by sharing solutions easily across the enterprise via browser-based data apps or services, a KNIME Business Hub feature. Data teams can quickly deploy KNIME workflows as data apps, designing them to include interactive capabilities for collaboration with line-of-business counterparts, as required, and share them via a link, or embed them into third-party applications.
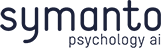
Symanto is a leading industry expert in Deep Learning, Natural Language Processing & Psychology at the forefront of AI development since 2010.