It was my great pleasure to host Dennis Ganzaroli in the first episode of the “My Data Guest” series, which was aired on September 22, 2021. In this conversation, Dennis shared some of the secrets and best practices that lead to becoming a successful data scientist: First among all, the passion for data as the most necessary tool to support your data science profession!
We also got to discuss some of his latest achievements, like the (correct) prediction of the final players in the UEFA Euro 2020 tournament or the (correct) estimate of the evolution of the COVID-19 spread all over the world. Last, but not least, we even got some recommendations on what to read to keep up to date with the constantly evolving field of data science.
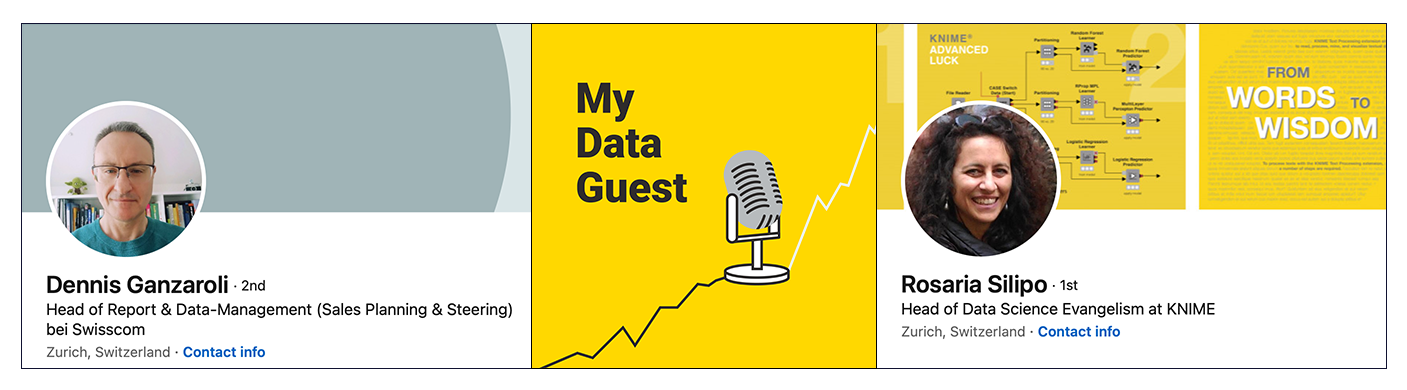
Dennis has been working with data for easily 20 years as a data engineer, data scientist, and sometimes as a data analyst. When you talk to Dennis you realize that his knowledge is much more than “just” his professional environment. His passion for data takes him further than his daily routine. Indeed, he applies classic and modern data science algorithms to predict COVID-19 spread or the winner of the UEFA Euro 2020 soccer tournament. The beauty is indeed this versatility, this ability to apply data expertise to every aspect of life. Data is data after all: Everything can be converted to numbers, inspected, and predicted.
Rosaria: Hi Dennis, tell us about your professional self and what you do in your job.
Dennis: I work for a big Telco in Switzerland as Head of Reporting and Data Management. We measure the performance of the sales channels and do everything from data integration and data blending through to creating dashboards, reports and so on.
Rosaria: Do you use KNIME in your everyday work?
Dennis: Yes. We use KNIME Analytics Platform mainly as an ETL tool and we also use KNIME Server to automate our workflows. We have a lot of daily reports that have to be ready in the morning, so we are happy to have such a solution. We also combine KNIME with other tools - mainly with Tableau. But I always say to the stakeholders: Tableau is just the car body, KNIME is the real engine.
Rosaria: Tell us about the biggest challenge you’ve had to solve in your professional life.
Dennis: The biggest challenge was, and will always be, explaining the story behind the data to clients and stakeholders. Data science is all about storytelling. You need strong communication skills and a good visualization of the data. As they say: “A picture is worth a thousand words.”
Rosaria: There is a high demand for data scientists in the employment market and yet data science is still not part of the traditional education system. People often have to learn skills themselves, sign up for online courses, and read the literature. Which books would you recommend to people who are wanting to learn new skills? You’re also writing your own book. Aren’t you?
Dennis: I very much like your book, Rosaria, about Codeless Deep Learning. It’s easy to understand and very useful. But yes, I just started writing my own book with the title “KNIME Solutions for Real World Applications“. It is a compilation of real world cases solved with KNIME together with other tools. I also read blog articles regularly to keep up to date. For example the journals Towards Data Science, Low Code for Advanced Data Science on Medium, and everything that can be applied in data science.
Note. Dennis Ganzaroli was Community Contributor of the Month in April 2021. In this program, KNIME recognizes community members who are doing unique and interesting things with KNIME software or sharing useful data science tips and tricks. Dennis was recognized for his blog articles, in which he shares experiences and best practices for analyzing data using KNIME software.
See more community members in the Contributor of the Month - Hall of Fame 2020/2021.
Rosaria: What is your advice for aspiring data scientists?
Dennis: Whenever somebody asks me this question I ask back: What are your hobbies? And if data science is not your hobby - you have to change hobbies! I think that “learning” alone is not enough. You must live it and love it to succeed.
Rosaria: What skills are most underestimated by candidates but a plus on the job?
Dennis: To keep cool in stress situations and never forget that it’s a job and not a game. So although I believe strongly that data science must become your hobby [if you want to be successful], the job is not a hobby. A lot of the time you will be doing things that you don’t like but that are still very important.
Rosaria: Let’s talk about how you’ve used your expertise with data outside of your professional life. On June 10, one day before the start of the UEFA Euro 2020 soccer tournament, you managed to correctly predict what the final game would be: England vs Italy. How did you do that?
Dennis: I asked Maradona :-) No, I used a fairly well known approach in the sports betting industry. I used a linear regression model to calculate the ratings of the teams.
Rosaria: But the model predicted that England would have been the winner.
Dennis: Not exactly, the model just calculated the power ratings of the teams before the tournament. So, England, Italy, and Spain were in the top three. By the way, all three teams made it to the semi-finals. Though Denmark was a surprise. Nobody saw that coming, not even the soccer experts. It’s important here to notice that soccer is a game with a lot of randomness. Scores are very sparse and a final match can be decided by a penalty shootout. Therefore, it’s not always possible to make a precise forecast. All in all, I think my power ratings were good, because the past games that I used as training data, reflected the strength of the teams well.
Rosaria: So, it was quite a simple model. No deep learning?
Dennis: Exactly, no deep learning, no strong GPUs, just a simple linear regression model.
The key factor here was to include domain knowledge. For example, the home field advantage is a very important factor in soccer. Even without spectators - it’s still there. Another point is that you have to take just the right portion of data - the portion that’s best to train the model. For example: after a big tournament, often the coaches change and the players change too. So it’s better to filter out those games happening right before such changes are made in the teams.
Rosaria: What about another project of yours where you predicted the spread of COVID19 worldwide and country by country?
Dennis: My motivation behind this project was to forecast the evolution of the COVID19 pandemic. In the beginning just for China and then for every country in the world. Yes, I wanted to answer the question: When will this pandemic be over?
Rosaria: Which model did you use?
Dennis: The evolution of a pandemic is like a growth process. At the beginning it’s an exponential function, then it changes to a sigmoidal curve. This is best described by a logistic function. However, then, when several waves followed, this simple approach does not work anymore and another approach is needed. I found out that Rockefeller University had already used a method called Loglet Analysis (also called wavelets) in the late 90s to forecast the evolution of multiple overlapping logistic functions.
Rosaria: Was this project with KNIME Analytics Platform or with Jupyter?
Dennis:I used KNIME Analytics Platform together with Jupyter. Indeed, you can call Python from KNIME. So the data was prepared in KNIME Analytics Platform but the loglet model was calculated in Jupyter with the scipy-package.
Rosaria: Thank you, Dennis, for this insight into your job and your other projects. How can data scientists in the audience get in touch with you or your work?
Dennis: I’ve written articles on Medium and I’m also posting some interesting stuff on Linkedin and Twitter. I have also created a Facebook group Data Science with Yodime. And on my Youtube channel you will find some interesting videos about Data Science, and not only. Of course all my workflows can be downloaded from my public space on the KNIME Hub.