The consecutive master's program in Controlling & Management at THM Business School, in Germany, is aimed at students who would like to holistically design the steering of medium and large-sized companies. Specialist competences in steering-relevant functions thus form an essential core of this program. This includes traditional disciplines such as strategic management, management accounting, and financial accounting. Recently, we added a course on business analytics to the curriculum, which is a modern essential for our program. This discipline acts as an interface between business administration and data science by supporting operational decision-making through data-based analytical models.
A major challenge in adding business analytics into the existing master's curriculum was the fact that the existing curriculum offers a very wide range of topics to provide students a holistic understanding. At the same time, this means that covering business analytics in several courses is not possible, unlike in more specialized master’s programs.
Four key aspects of KNIME helped me deal with this challenge:
First, students do not have to learn code like Python or R to get started, and can quickly get into actual analysis with KNIME’s code-free software.
Second, the graphical user interface of KNIME, along with its visual workflows, support the students in their procedural understanding.
Third, KNIME offers a fully functional version of the software free of charge, which can be used in all common operating systems and, thanks to a large variety of interfaces, can be integrated into a wide range of IT landscapes (and companies).
Fourth, KNIME includes a wide range of teaching resources (online courses, publications, community forums, etc.), which students can use to continue their education even after completing the module.
My teaching concept and course roadmap
The course on business analytics, spread over seven days, roughly divided into two parts.
In the first part, students learn the basics of business analytics and how to use KNIME on-site in a computer lab on the THM Campus.
In the second part, students work in teams to develop solutions for three practical business analytics cases: data processing for a mergers & acquisitions deal, forecasting a model for customer churn, and forecasting of real estate market development. Students then present and discuss their solutions through a management lens. While the work on the second part starts on-site, the teams continue working on solutions with their own laptops remotely. The final presentations are also conducted online.
In more detail, the course agenda is as follows:
- Day 1: Conceptual introduction to business analytics and introduction to KNIME
- Day 2: Selected machine learning methods and their application in KNIME (with a focus on decision/regression trees and linear regression), which includes a guest lecture by KNIME
- Day 3: Deep dive into time series analysis methods (with a focus on SARIMA)
- Days 4 and 5: Teamwork on business analytics case studies (with coaching)
- Two-week break (approximately), during which students continue working on the case studies independently
- Days 6 and 7: Case study presentations and discussion
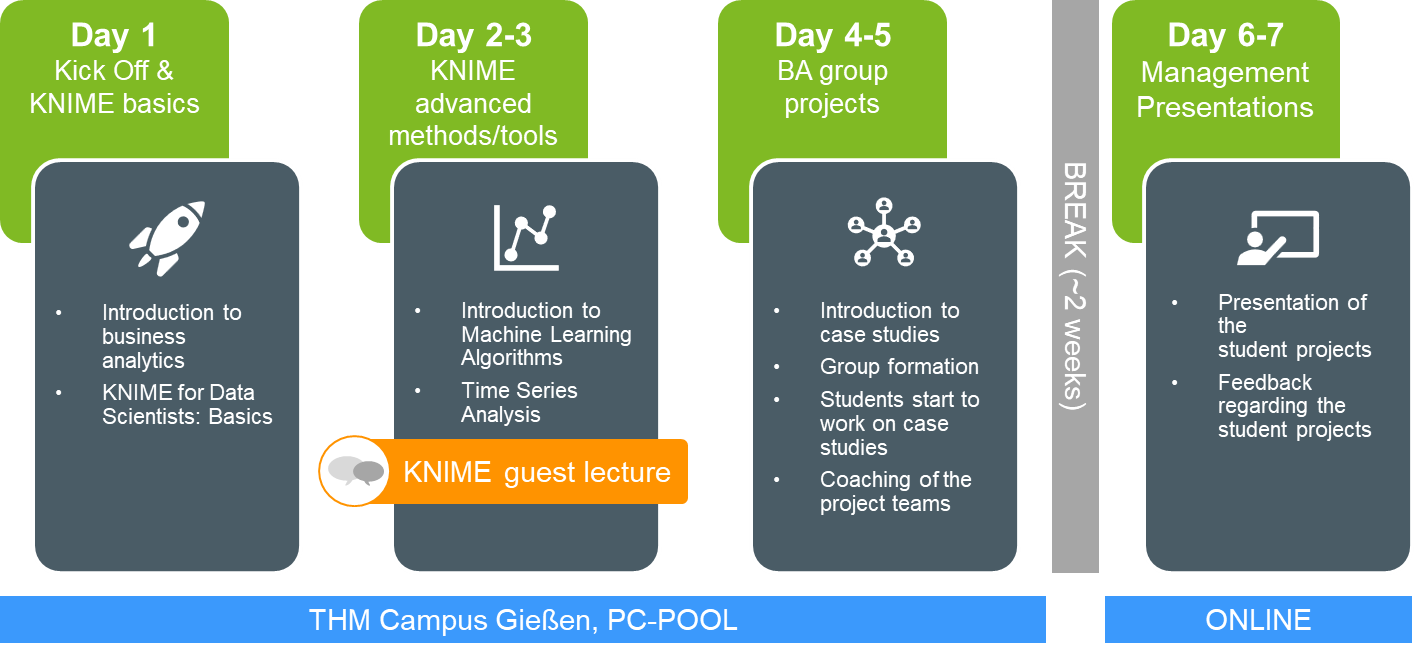
The teaching materials are derived from official KNIME course slides, which are thankfully available to me as a member of the KNIME Educators Alliance. From the official course materials, I picked the concepts and tools which suited my teaching focus best and, in a few cases, I added more theoretical and conceptual elements. The materials stem from the following KNIME courses:
- KNIME Analytics Platform for Data Scientists: Basics (L1-DS)
- Introduction to Machine Learning Algorithms (L4-ML), with emphasis on decision/regression trees and linear regression
- KNIME Introduction to Time Series Analysis (L4-TS), with emphasis on ARIMA and SARIMA models
I selected these topics and methods to provide students with a good starting point for future business analytics activities. Time series analysis might seem to be very specific for such a general approach, but I included it because it is a paramount tool for financial forecasting.
My view on business analytics and its implementation with KNIME focuses mainly on financial and managerial use cases. I am glad that my teaching is supplemented with a guest lecture by Dr. Stefan Helfrich from the KNIME Educators Alliance team. He broadens the students’ understanding of business analytics by introducing them to a variety of different use cases. Moreover, he provides them with an overview of the breadth of training opportunities that are available for KNIME.
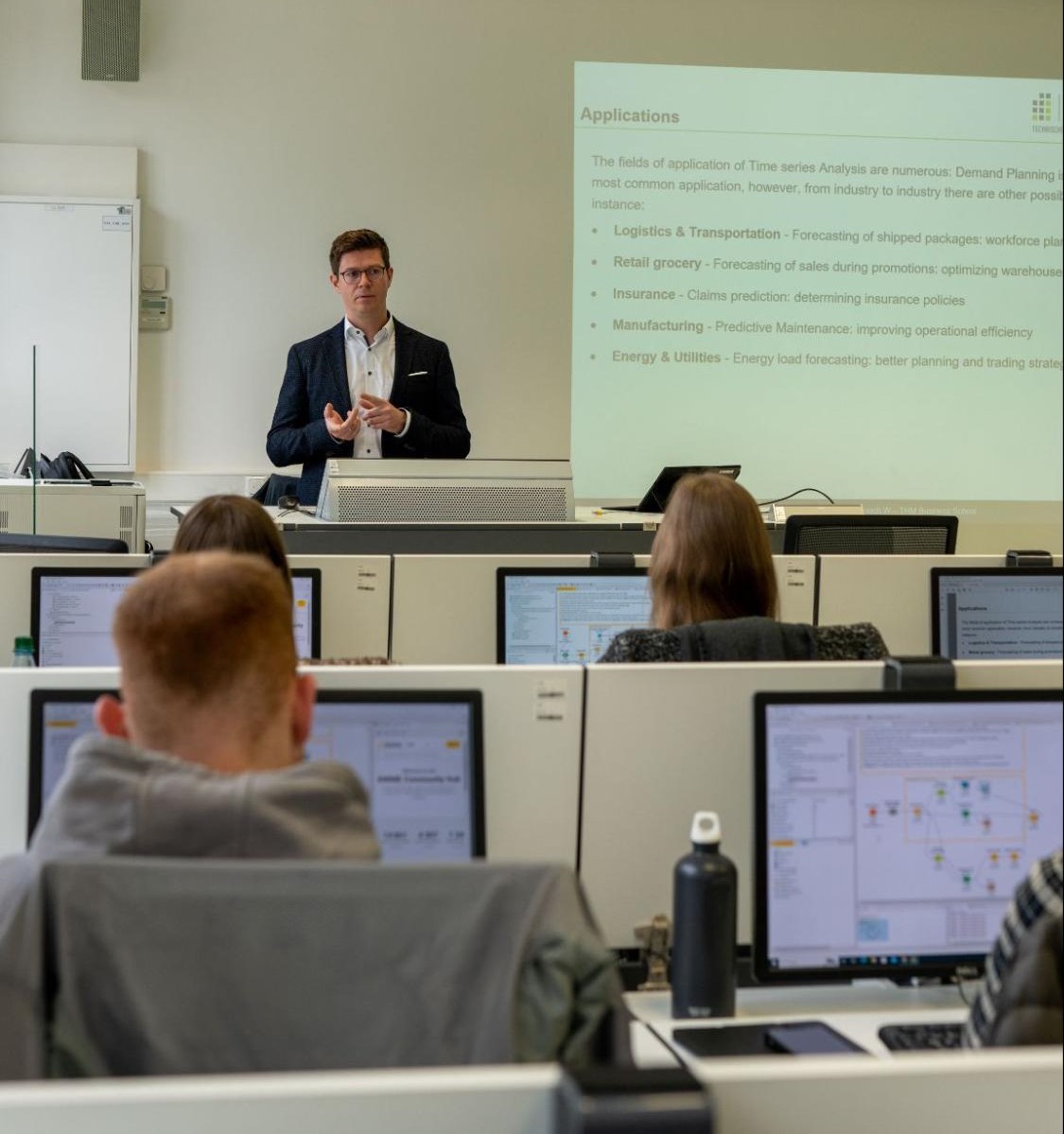
5 key takeaways
I was able to gather the following key takeaways for future courses:
- Practice makes perfect (and helps understanding)
When I first taught the course, I placed more emphasis on presenting and explaining the relevant concepts to students than on letting them practice with KNIME on their own. This quickly changed as I realized that the exercises with KNIME were at least as important as my explanations, since for many of the students, their own experimentation was crucial to a deeper understanding. I shifted the time allocation during the module in favor of the practical exercises.
- Helping students to help themselves is better than giving instructions
At the beginning, I often gave students direct solutions to questions in the practical exercises (e.g. "Please tick this box in the options menu."). However, this limited the students' own experimentation, and thus their learning progress,considerably. Better were vague statements (e.g. "Perhaps take another look at the configuration options.") or references to the online learning resources. The latter also had the advantage of allowing students to become directly familiar with the large variety of training options.
- One goal can have many paths
Of course, I already have my own solutions "in the drawer" for both the practical exercises and the case studies. However, when I see the students' solutions, I am always surprised by their creativity: different students or groups often come up with the same, correct solutions using completely different approaches. As a lecturer, this means that I have to stay flexible whenever I assess my students’ work. I always have to find my way into their specific logic.
- Adopting a management perspective at an early stage is crucial
At the end of the course, students present their case studies to a fictitious general management team. It is important to present the results in a generally understandable form rather than in technical detail, as the focus is on the practical implications for the company. Here, students who take a business perspective to their models or results at an early stage are the ones who are successful. When coaching students, I sometimes have to enforce this perspective by always getting back to the implications for the business.
- Sharing the workload but ensuring teamwork
The case studies at the end of the module are particularly labor-intensive, and most students split up the workload. It is essential that sharing the workload does not lead to individual students working on their models completely detached from their team. Otherwise, this leads to a lack of compatibility with the overall solution and, nearly always, has a negative impact on creativity. Many teams are successful by discussing the individual work steps together at regular intervals (with both a data-based and a business perspective) and integrating them into the overall solution.
As I have only recently included KNIME into my teaching portfolio, these five takeaways are only the start. With each course, I’m gathering more insight, which will help me adjust my teaching concept accordingly. I’m happy to say that positive student feedback to date confirms: “The course has real potential for future careers. It offers considerable added value by giving me the opportunity to learn essential new skills.”