Manufacturing value chains are complex. Goods produced in manufacturing plants are made up of multiple individual parts, and, depending on the product, the number can range from a handful, to several hundred or more. In the automotive industry for example, each car consists of thousands of individual pieces, which are produced and assembled in a multi-step production process. This large number is made up of not only what is in the manufacturing plants themselves, but also the entire supply chain and their multi-step plants.
In manufacturing, large volumes of traceability data are stored to be able to re-construct which parts were built into which products. Once implemented, traceability can have transformational impacts for the business. For example: if a faulty part or process issue is detected, manufacturers can trigger a product recall quickly and efficiently with the precise products batches that have been affected, radically reducing the amount of time and money spent on firefighting.
What is Traceability?
Traceability is the ability to track raw materials, work-in-progress, and finished goods through a manufacturing process using data. Data points can be linked from various steps in the process, resulting in an end-to-end system, where it becomes easy to identify which batch of raw materials from which supplier ends up in which final product and vice versa – see Figure 1 below.
At each step, shop-floor IT systems record the serial or batch identifier of the raw materials built into the sub-assembly. This isn’t the part number according to the bill-of-material — it’s the unique identifier of a physical object (typically a Data Matrix Code (DMC)). Due to reasons of size or economy, these objects are not necessarily equipped with individual IDs, but rather batch IDs. Large volumes of traceability data are created and stored so that it becomes possible to reconstruct an entire process if necessary. For example, identify which capacitor was built into which electronics control unit subassembly and ultimately into which vehicle.
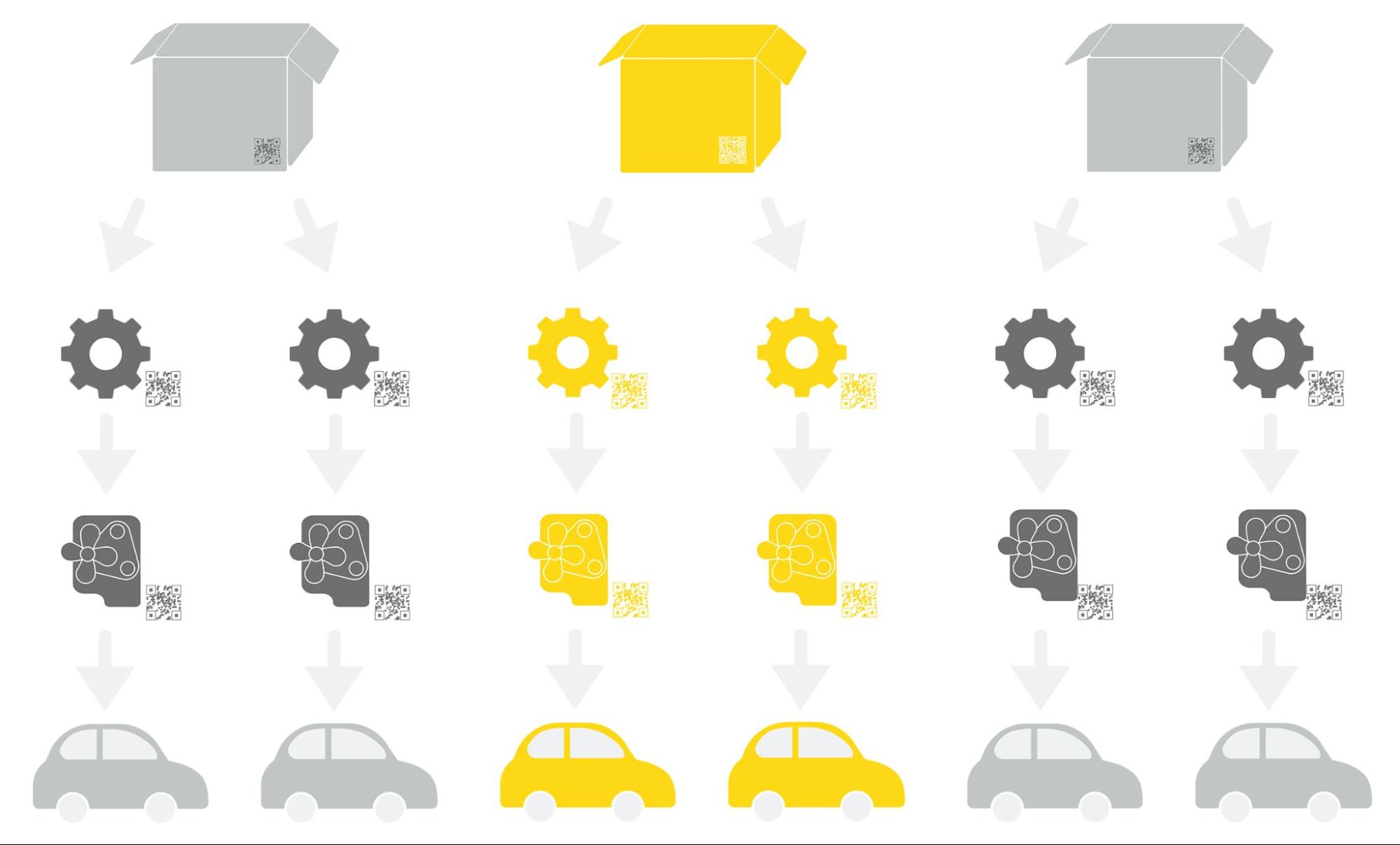
Modern product traceability provides a valuable digital mechanism for a thorough understanding of the production process, absolute control of quality, effective management, and debugging of complaints, damaged products, inefficiencies in production, and distribution of responsibilities.
The question is: what is the ideal technology setup to tackle traceability?
Traceability has Two Important Elements:
-
Firstly, storing traces: Designing a system that captures data from every production step marrying two parts
-
Secondly, querying specific time frames or batches and resolving multi-step production processes from every raw material to the final product
Standard production IT systems can reveal traceability links either by individual part reports or small batch analysis. But a bulk analysis covering thousands of parts or products is rarely available to business users from departments such as Quality Control, Industrial Engineering, or Research & Development. A sad state of affairs, given that a bulk analysis is often exactly what business users really need.
For example, a supplier quality specialist analyzes use cases on the impact of an electronic part on final product quality. The point at which analytics typically gets stuck is the link between the raw material data matrix code (DMC) and the final product identifier. As straightforward as it sounds, a simple mapping table between these codes is the missing piece of wisdom.
The IT department could help with some SQL and database magic, but this method is inefficient. Whilst data capturing typically happens in a single system, consistently, and in real time, the challenge is combining these individual pieces to create the big picture.
Serve Up Traceability Data via Self-Service Analytics
As mentioned above, one of the biggest traceability challenges is that it’s often only something IT can do in bulk. There is typically no self-service access for bulk traceability results.
A single system can give business analysts access to what they need and keep IT happy because they only have to set up the system once. An architecture with a backend for processing raw production data with graph analytical capabilities and a frontend embedded in a self-service analytics tool to allow consumers to seamlessly add their own analytical logic if and when needed, can solve this challenge.
KNIME Server can continuously process incoming production data items of the form “raw material A was built into sub-assembly B”. Pre-processed traceability results are then fed into the users’ KNIME Analytics Platform workbench. Users access the results via a pre-configured data application, which exposes them to the data they need and, depending on the configuration, allows them to run their own traceability analysis. In other words: IT builds or buys the data application once and business analysts can then access the data and continue building solutions - including adding their own business logic.
The advantage of this architecture is that the streaming data and heavy processing are handled by the backend on a continuous basis. The computational burden for the user on the frontend is significantly lower as only certain sections of the entire production data realm need to be loaded. The frontend can therefore run on a standard laptop PC of the business user - making the solution available to anyone with KNIME installed locally on their computer.
Reusability and Automation Removes Repetitive Work
Traceability is often the missing link in manufacturing data analytics use cases – as highlighted by the above supplier quality specialist example. Imagine two measurement files are available: One for the final product, and the other for a component or sub-assembly. Each has a column for a serial number (DMC), but these don’t contain matching values per se. Only the traceability mapping table depicted above relates the raw material to the product.
Tasks like this are common, not just in traceability but also in other manufacturing use cases. Users complete the same, repetitive tasks on a daily basis often reinventing the wheel each time. With the traceability component in the following workflow (Fig. 3), tasks such as a correlation analysis become trivial and can be easily automated. A business analyst only needs to:
-
Join the traceability mapping component to one of the data sources
-
Join this data with the other measurement file as the identifiers are mapped
-
Analyze the results — for example correlations of the raw materials’ production parameters to end-of-line quality parameters of the final product
Mass Data Traceability Opens the Door to Other Manufacturing Use Cases
Manufacturing is always going to consist of hundreds of processes and will continue to be data heavy. That’s why COOs and CIOs should consider a data analytics tool to not only ease the burden of IT teams but enable business analysts to do more with the data.
There are many manufacturing use cases where data analytics outcomes can be boosted by traceability. Quality engineers can proactively analyze raw materials’ effects on product quality. Suppliers can be compared based on sub-assembly production parameters or results. A risk management use case could simulate the effect of troublesome raw material production batches to determine, for example, “how many cars are going to be affected if a part is found to be defective post-shipment?”
When combined with subsequent supply chain data, information transparency is maximized in case of real quality issues. With one click it’s easy to identify where each part is and how many of the bad parts are still in the warehouse, currently in production, assembled into the product but not yet delivered, or already shipped. And in firefighting mode, answers can be provided much faster and more reliably, which is arguably the most important feature of traceability.