What is it about data science that puts people off learning it?
Despite huge investments in data science initiatives, 94% of organizations face a list of challenges that prevent them from developing and implementing the data science projects that are needed to improve operational efficiency. Top of the list is a lack of skilled talent.
And here’s the thing: This lack won’t be filled simply by having more data scientists coming out of universities. The degree in data science proves a baseline qualification in that specific field, but a lot of organizations aren’t only looking for “pure” data scientists. To implement more data science projects, companies also need their existing workforce to gain data science skills. That’s because these people already have valuable on-the-job experience and business understanding that enables them to recognize when something in the data looks off.
With data science skills, you, the supply chain specialist, will be able to derive more data-driven insight because you can integrate your 10+years of experience. You, the accountant, will be able to automate all the repetitive data analysis tasks, freeing up time to apply your specific expertise to more value-adding activities. You, the content marketer, will be able to quickly apply analysis results to your planning to run sophisticated, highly targeted campaigns.
As well as the promise of contributing meaningfully to cutting-edge projects, acquiring data science skills opens up prospects of career advancement, recognition, and salary increases. And yet the data science talent shortage persists.
What’s putting people off? Well, it’s just a couple of misconceptions.
Essential data science isn’t just for scientists
One of the most common misconceptions is that data science is only for, well, scientists – people with advanced degrees and strong technical backgrounds. But data skills will soon be just as expected by employers as say skills in Word, Excel, and Powerpoint.
Universities are responding to this trend by introducing data science modules across the spectrum to provide students from multiple disciplines the opportunity to gain what is increasingly seen as a modern essential.
And increasing numbers of professionals have data science upskilling on their radar. Professionals who you might not normally associate with “doing” data science, for example farmers, social scientists, healthcare workers, content marketers, entire football associations, and many more, are signing up for data science courses.
Any professional can develop valuable data science skills.
Essential data science isn’t just for coders
The biggest entry barrier to data science upskilling is user-friendliness of the tool. One of the most common data science myths is that you first have to learn to code. But coding is just one way to do data science. And you don’t have to learn to code, if you don’t want to.
You can get started with data science techniques through an easy-to-use interface with no-code/low-code data science. Here, you’re building your data analysis by drag and drop. In contrast to coding approaches, the graphical user interface lets you concentrate on the data science without having to learn all the scripting instructions first.
Low-code “lowers the entry barrier to the world of data, and enables fast developments with satisfying and valuable results,” says Jörg Endter, who teaches data science at the German Chambers of Industry and Commerce (IHK). “Participants don’t need previous programming experience, just an affinity for data.”
The cherry on the top is when the low-code data science tool is open source and free to use.
Essential data science isn’t just for people with time
Picture being able to run your financial reporting at a click, produce a visualization in a minute, import data without constant error messages.
When you start learning the basics of data science you start thinking about the process your data goes through (as opposed to thinking about a static table of data). As you build out the data process – assembling a sequence of logical steps your data goes through – you’re starting to create a re-useable process, reducing rework, and freeing up time.
With a shift in mindset from the data table to the data process, you’ll identify multiple tasks that can easily be automated, gaining you savings of over 5 weeks per year of manual work. Gaining data science skills isn’t done overnight, but the return on investment makes it worthwhile.
Get a head start on your learning by seeking out resources shared by the open source data science community. This community is known for its supportive, collaborative spirit, creating and sharing solutions, tools, and resources. Instead of starting with a blank canvas, you can access a huge library of knowledge that’s open for anyone to learn from and contribute to.
The easier it is to access resources, the easier it becomes to learn and build solutions yourself. With each additional resource, shared by friends and colleagues, your learning gains momentum. It’s the data science upskilling flywheel effect!
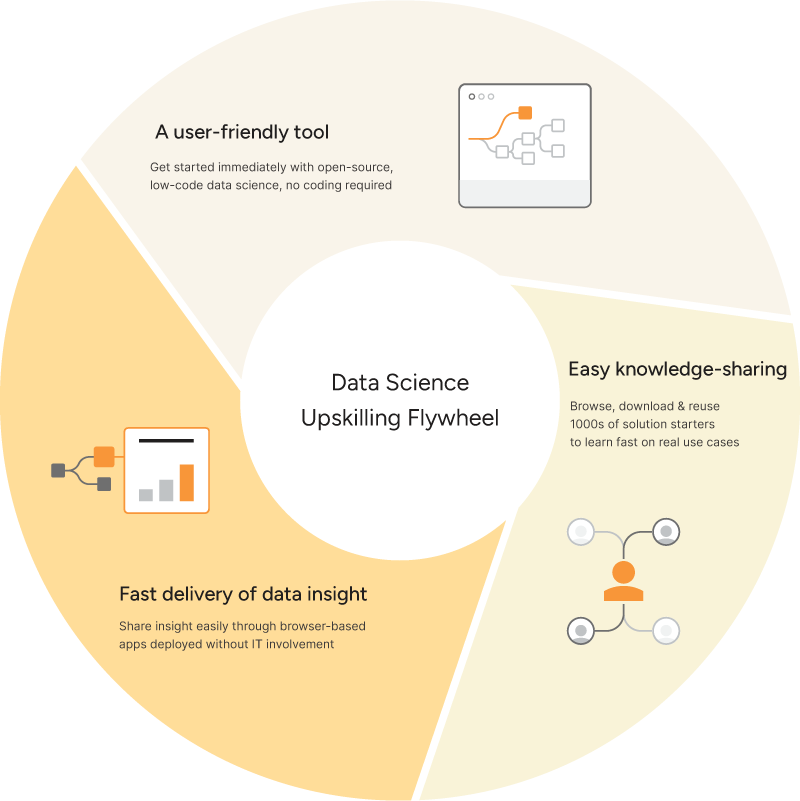
And the return on investing your time isn’t just about efficiency. With the skills to apply more advanced data science techniques, you’ll improve the quality of your work, tapping into insights you didn’t know existed.
Pair up your skills with data science techniques
The field of data science offers a huge toolbox of techniques. The techniques behind AI, robots, and chatGPT, for example, fill just one compartment. In the data science toolbox, you’ll also find techniques for storing and collecting data, making different types of data comparable, exploring data, and creating charts and graphs to explain the data.
The data analyst, for example, draws on specific data science techniques to organize, examine, and visualize data to find patterns, answer specific questions, and explain what the patterns mean by building specialized reports. The data scientist will apply more advanced data science techniques to dive a little deeper into the data, to not only find patterns, but predict future outcomes based on the data.
As you gain knowledge of different techniques you can decide for example, when a simple statistical test or a chart might tell us more useful things than a complex machine learning model. As you build out your analysis, your domain expertise is your compass, informing you when results feel slightly off, when you need to try a different technique, select a different plot to visualize the analysis, and more.
Your business understanding paired up with the ability to apply the best data science techniques makes you the skilled talent everyone is looking for.