Everything is “data-driven” these days. Data-driven marketing, data-driven management, data-driven business decisions — information is truly power for organizations.
And to harness the full potential of data, businesses need to understand what’s called the data lifecycle — the stages that data passes through.
The data lifecycle is comprised of eight stages. We’ll explain each one, who is involved, and provide concrete examples through the lens of a sample project involving customer sentiment analysis.
In this sample project, Company X wants to analyze customer sentiment from social media to improve its customer service approach.
What is the data lifecycle?
The data lifecycle encompasses a series of eight stages through which data passes — from its creation to its end use in decision-making. Each stage involves specific processes and stakeholders that ensure data is properly managed, analyzed, and utilized.
Understanding the data lifecycle helps organizations optimize their data-handling practices. This leads to better data quality, improved security, and smarter business decisions. By effectively navigating these eight stages, organizations can transform raw data into information they can really use to drive innovation.
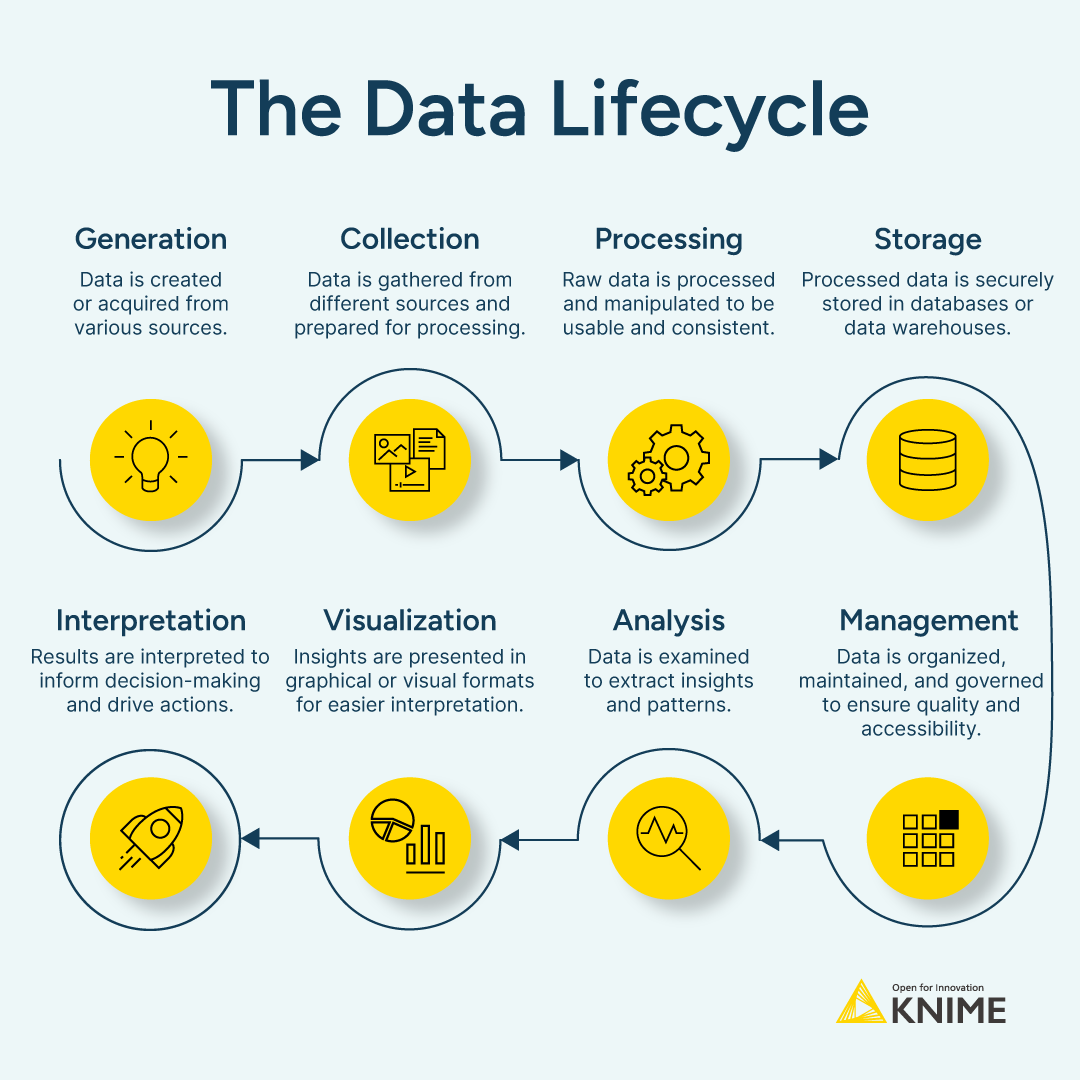
What are the 8 stages of the data lifecycle?
The data lifecycle can be broken down into eight distinct stages, each of which plays a vital role in transforming raw data into valuable insights. Understanding these stages helps organizations streamline their data processes — which helps ensure efficiency, accuracy, and security.
1. Data generation
Data generation marks the birth of the data lifecycle. This first stage involves the creation of data from a variety of sources, including:
- Customer interactions
- Business and financial transactions
- Social media activities
- Internet of Things (IoT) devices
For example, a retail company might generate customer data from point-of-sale (POS) systems, e-commerce shopping carts, and feedback forms.
Who is involved in data generation?
The main roles typically involved in data generation include:
- Data engineers: Develop systems and execute processes for generating data.
- IT staff members: Build and maintain the technical infrastructure that supports data generation.
Company X example:
Data generation occurs when customers like or comment on their posts or mention them on social media.
2. Data collection
The second stage in the data lifecycle, data collection, involves the structured gathering of relevant data from a variety of sources like:
- Surveys and questionnaires
- Web scraping
- IoT sensors
- Application programming interfaces (APIs)
- Transaction records
- Social media monitoring
- Observations
This stage is critical to the process, as it ensures that the data needed for analysis is accurately aggregated and data loss is reduced.
Who is involved in data collection?
The main roles typically involved in data collection include:
- Business stakeholders: Ensure the data they need for decision-making is being collected.
- Data engineers: Integrate data from various sources into centralized databases.
Company X example:
Data collection occurs when the business uses web scraping tools to collect data from social mentions and integrates it with customer purchase data collected via e-commerce platforms.
3. Data processing
Data processing is the third stage in the data lifecycle. It involves the following steps that prepare data for analysis:
- Data cleaning: Removing duplicate content, correcting errors, and filling in missing values.
- Data transformation: Converting raw or unstructured data into a suitable format or structure.
- Data integration: Combining data from disparate sources into a cohesive dataset.
- Data reduction: Simplifying datasets by eliminating redundant or irrelevant data.
- Data validation: Ensuring processed data meets organizational standards and accurately reflects its original sources.
These steps prepare collected data for meaningful analysis, ensuring accuracy and consistency.
Who is involved in data processing?
The main roles typically involved in data processing include:
- Data engineers: Develop ETL (Extract, Transform, Load) pipelines to automate processing.
- Data scientists: Explore raw data to determine useful sources and formats, informing the creation of pipelines.
Company X example:
Data processing occurs when the business processes its social media data by removing repeated posts, comments, or identical reviews posted on different platforms. It also includes correcting inconsistencies in usernames or hashtags and standardizing date formats.
4. Data storage
The fourth stage of the data lifecycle, data storage, is essential for ensuring data is accessible, safeguarded, and backed up for future use. This stage focuses on data privacy — configuring your storage solution for privacy — by securely storing processed data in:
- Databases
- Data warehouses
- Cloud storage solutions
- Data lakes
- On-location storage (e.g., physical servers)
This stage in the data lifecycle involves choosing the right storage solution for your data protection needs and organizing data for efficient retrieval and use.
Who is involved in data storage?
The main roles typically involved in data storage include:
- Database administrators: Manage data storage systems.
- IT staff and security teams: Ensure data security and backup protocols are in place.
Company X example:
Data storage occurs when the business securely stores social engagement data like comments, captions, and reactions in cloud-based data warehouses. This enables easy access for analysis while facilitating scalability.
5. Data management
Data management is the fifth stage in the data lifecycle. It encompasses the ongoing organization and maintenance of data through:
- Data governance: Establishing standards, defining user roles, and ensuring compliance. Setting policies for data sharing across departments.
- Data quality management: Monitoring, cleaning, and validating data.
- Data security: Implementing encryption and access controls and conducting security audits.
- Data access and retrieval: Setting up and using indexing and cataloging techniques.
- Data integration: Creating a unified view of data and ensuring consistency.
- Data archiving and deletion: Caching or deleting outdated or infrequently used data.
These processes ensure data remains accurate, accessible, and meets regulatory requirements. And, most importantly, ensures privacy while data is being used.
Who is involved in data management?
The main roles typically involved in data management include:
- Data engineers: Facilitate better decision-making by ensuring data is secure, accurate, and accessible.
- Database governance and security teams: Implement policies and data standards. Maintain data privacy.
Company X example:
Data management occurs when the business puts policies in place that ensure customer data from sources like Facebook and Instagram is handled securely, regularly cleans and validates it, and archives old interactions.
6. Data analysis
Data analysis, the sixth stage in the data lifecycle, is where real value is discovered by using analytical tools and techniques to identify patterns, trends, and correlations in data. The key components involved are:
- Descriptive analytics: Summarizes past data to help organizations understand what has happened.
- Diagnostic analytics: Examines data to determine why certain events or issues occurred.
- Predictive analytics: Uses historical data and machine learning (ML) to forecast trends and future outcomes.
- Prescriptive analytics: Guides future actions by predicting optimal steps to reach a specific goal.
This stage makes it possible to extract meaningful insights from data so businesses can make more informed decisions.
Who is involved in data analysis?
Perhaps obviously, the main role involved in data analytics is a data analyst, who oversees some of this work. For more advanced tasks around predictive and prescriptive analytics, a data scientist is usually involved. Business stakeholders will also be included in data analysis processes so they’re able to ask questions and provide information about company goals. Other roles typically involved in data analysis include:
- Data analysts: Take on most data analysis tasks. For more complex tasks involving machine learning, they would rely on a data scientist.
- Data scientists: Facilitate better decision-making by ensuring data is secure, accurate, and accessible, and doing advanced data work like predictive and prescriptive analytics.
- Database governance teams: Implement policies and data standards.
Company X example:
Data analysis occurs when the business uses natural language processing (NLP) techniques to analyze social media sentiment and identify common themes in customer feedback. This allows Company X to create more targeted marketing campaigns.
7. Data visualization
The seventh stage of the data lifecycle is data visualization. It involves representing data graphically to communicate data insights effectively. This is the stage in which complex data becomes more understandable through visualizations like:
- Charts and graphs
- Interactive and real-time dashboards
- Geospatial maps (e.g., heat and choropleth)
- Advanced techniques like scatter plots, histograms, and tree maps
Through graphical representations, this stage makes data understandable for organizational stakeholders and allows them to take action confidently.
Note: Although data visualization is the 7th step in the data lifecycle, a data analyst, data scientist, or data engineer, will likely refer to multiple types of visualizations in the exploratory stage of their analysis and perhaps even earlier in the process.
Who is involved in data visualization?
The main roles typically involved in data visualization include:
- Data scientists: Develop intricate visualizations to illustrate analytical models and outcomes and ensure they accurately reflect insights and trends.
- Business analysts: Use visualizations to present findings to stakeholders in an understandable format.
Company X example:
Data visualization occurs when the business creates interactive dashboards that illustrate metrics like social shares, comments, and follower growth over time and heat maps that show regional social engagement levels across different locations.
8. Data interpretation
Data interpretation is the final stage in the data lifecycle. This is the stage in which the analyzed and visualized data is used to make informed business decisions. The key activities involved in this stage include:
- Reviewing dashboards, charts, and graphs to identify key insights.
- Making sense of analytical results and drawing conclusions to explain business performance.
- Suggesting actions based on data findings and providing strategic guidance on marketing, product development, and customer engagement.
- Presenting findings and using storytelling techniques to convey the significance of data insights.
This stage is important to an organization’s data usage practices, as it ensures that insights derived from data analysis and visualization are effectively utilized to drive strategic decisions and improve outcomes for an organization.
Who is involved in data interpretation?
The main roles typically involved in data interpretation include:
- Business analysts: Use visualizations to present findings to stakeholders in an understandable format.
- Stakeholders and executives: Make tactical decisions based on data.
Company X example:
Data interpretation occurs when business executives use the visualized social media data to refine customer service strategies and enhance overall customer satisfaction. They do this by focusing on areas with negative customer sentiment.
Why is the data lifecycle helpful?
Understanding the data lifecycle and the data lifecycle management (DLM) process is essential to organizations for several reasons:
Efficient data management
Each stage of the data lifecycle ensures that data is handled properly, which reduces errors and enhances data quality for organizations. Structured processes allow for systematic data collection, storage, and maintenance, which reduces inaccuracies and inconsistencies and protects sensitive data.
Improved decision-making
Structured data processes lead to more reliable insights. By following a clear lifecycle, organizations can trust and use data that is relevant and accurate, which is vital for making informed strategic choices.
Regulatory compliance
Managing data and its deletion properly means ensuring compliance with security and privacy regulations, which mitigates risk for organizations. By adhering to these lifecycle stages, businesses can maintain audit trails, enforce data governance policies, and confirm that data handling practices meet legal requirements.
Resource optimization
Streamlined data processes save organizations time and resources, which improves overall business efficiency. Automating data handling tasks and maintaining well-organized data systems reduces the time and effort it takes to manually process data and correct errors.
Data consistency and reliability
Maintaining consistency in data handling makes data trustworthy, but in reality data sources or pipelines can change year over year, making it hard to compare apples to apples. Maintaining consistent data sources and pipelines is crucial for conducting accurate analyses and deriving insights that organizations can actually use in the long-term.
Enhanced collaboration
In order to promote effective communication and collaboration across teams and departments, everyone must understand their job within the lifecycle. When clear roles and responsibilities are established at each stage, it facilitates better teamwork and project coordination.
Scalability and flexibility
A well-defined data lifecycle allows organizations to scale their data operations efficiently as their data needs increase. It also provides the flexibility to adapt to new data sources and technologies, which helps to future-proof organizations’ data management strategies.
When organizations understand and implement the data lifecycle, they can optimize their data handling practices. This can lead to more comprehensive and effective data utilization, better customer retention, increased ROI, and a stronger competitive advantage.
Data Lifecycle FAQ
Here are a few frequently asked questions and answers about the data lifecycle.
What is the First Stage of the Data Lifecycle?
The first stage of the data lifecycle is data generation. This is the stage in which data is created within various sources.
Why is Data Processing Important?
Data processing is important because it ensures that raw data is cleaned, transformed into suitable formats, and organized properly so it’s ready for accurate analysis.
What are the 5 Stages of the Data Lifecycle?
Although different organizations combine certain steps and list different numbers of data lifecycle stages, we define it within eight stages:
- Data generation
- Data collection
- Data processing
- Data storage
- Data management
- Data analysis
- Data visualization
- Data interpretation
What Do You Mean by Data Life Cycle?
The data life cycle is an 8-stage process that guides the creation, management, analysis, and utilization of data to ensure its accuracy, protection, and usefulness in decision-making.
Learn More About the Data Lifecycle
The data lifecycle is a comprehensive framework that guides the management and informed use of collected information, from data creation to its final utilization in business decision-making. By understanding and effectively implementing each stage, organizations can unlock their data’s potential and put it to work for them.
KNIME Analytics Platform supports each stage of the data lifecycle and can make data management and interpretation more accessible and efficient for businesses of all kinds.